Extended reality (XR), an umbrella term encompassing virtual and augmented reality (VR/AR), provides immersive and safe simulated environments to train and test both humans and cyber physical systems (CPS). In this page, we share related work on Scenic in XR, or in short, ScenicXR.
Task Distribution Aware Psychomotor Skill Training with Probabilistic Programs and Bayesian Knowledge Tracing in Virtual Reality
Probject Abstract: Virtual reality (VR) is used to train psychomotor skills for domains both within VR, e.g. games, and beyond VR, e.g. sports and healthcare. Although it is a common practice to employ variations of tasks to train psychomotor skills, how to algorithmically predict psychomotor skill acquisition given the task variations, or a distribution, has not been investigated. To address this problem, we derive and adapt ideas from intelligent tutoring systems (ITS), a sub-field of learning sciences. We formally model and generate task distributions with physical constraints that are designed by instructors using a probabilistic programming language. We investigate the effectiveness of Bayesian knowledge tracing (BKT) from ITS to predict psychomotor skill acquisition. Our algorithm sequentially sample a task from a probabilistic program, generates it in VR, and updates the BKT prediction using the performance of a user on the task. We conduct a between subject study that compares BKT to self-prediction of skill acquisition. Our study shows that the experimental condition outperforms the control, and BKT contributes to much more consistent learning outcomes than self-prediction.
Modified Fugl Meyer Assessment in Augmented Reality
Probject Abstract:
Objective: Assess the feasibility of the ARPA use for patients with a history of stroke affecting the upper extremity.
Background: Over 700,000 patients in the US suffer from stroke yearly and about 70% of patients with stroke will experience some degree of arm weakness. Despite the importance of rehabilitation, access to outpatient rehabilitation is often limited by long waitlists and by the need for appointments in person. Home-based rehabilitation is an alternative to increase access. However, methods to accurately track patient progress at home are needed by clinicians to create the plan of care.
Design/Methods: Based on the original Upper Extremity Fugl-Meyer (FMA-UE) assessment, we developed a virtual version of the FMA-UE (vFMA-UE) with 21 tasks excluding reflexes, implemented using our customized assessment software and an augmented reality headset. The primary outcome of the study was to assess patient tolerance and experience. Two patients with prior stroke underwent an ARPA followed by a standard FMA-UE evaluation by an occupational therapist or physician. Software calibration was allowed between each patient.
Results: Two patients participated in the initial evaluation. Both patients completed the augmented-reality assessment and reported a positive experience. The first patient scored 37/57 on vFMA-UE and 39/60 on FMA-UE, while the second patient scored 40/57 and 41/60, respectively. Both patients indicated that breaks during the assessment are required. One patient required assistance from another person to adjust the headset, but neither patient required assistance to start the application or complete the assessments.
Conclusions: In conclusion, based on our preliminary results, our ARPA system successfully delivered a virtual assessment of upper-extremity deficits in patients with stroke, suggesting its feasibility. In the next phases, we plan to assess the correlation between vFMA-UE and FMA-UE with a larger group of patients, demonstrate the accuracy of the system and deliver rehabilitation remotely.
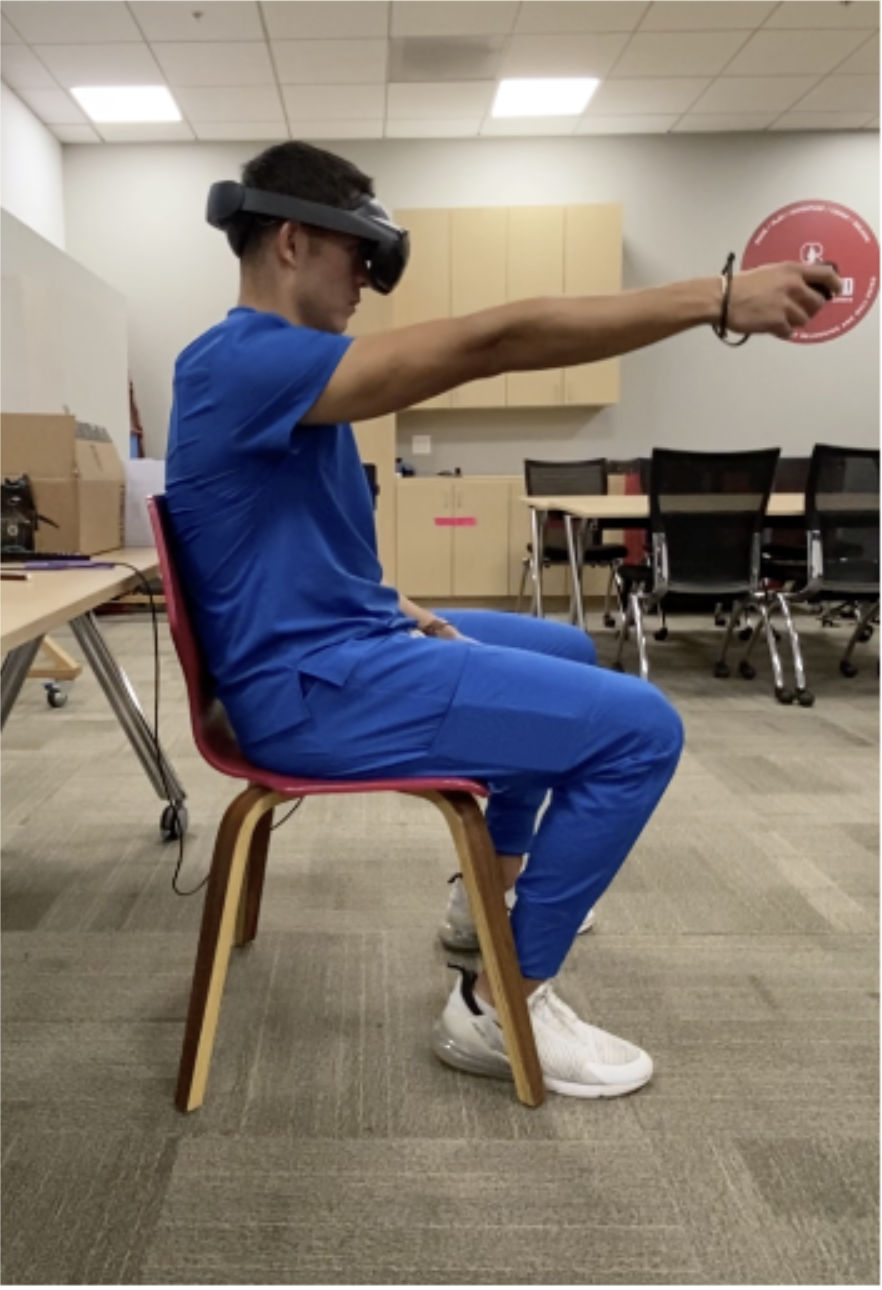